Tutorial 5: Lagged regressors#
Lagged regressors are used to correlate other observed variables to our target time series. For example the temperature of the previous days might be a good predictor of the temperature of the next day.
They are often referred to as exogenous variables or as covariates. Unlike future regressors, the future of a lagged regressor is unknown to us.
At the time \(t\) of forecasting, we only have access to their observed, past values up to and including \(t − 1\).
First we load a new dataset which also contains the temperature.
[2]:
import pandas as pd
# Load the dataset for tutorial 4 with the extra temperature column
df = pd.read_csv("https://github.com/ourownstory/neuralprophet-data/raw/main/kaggle-energy/datasets/tutorial04.csv")
df.head()
[2]:
ds | y | temperature | |
---|---|---|---|
0 | 2015-01-01 | 64.92 | 277.00 |
1 | 2015-01-02 | 58.46 | 277.95 |
2 | 2015-01-03 | 63.35 | 278.83 |
3 | 2015-01-04 | 50.54 | 279.64 |
4 | 2015-01-05 | 64.89 | 279.05 |
[3]:
# Optional:To align the scale of temperature with the energy price, we convert it to Farenheit:
df["temperature"] = (df["temperature"] - 273.15) * 1.8 + 32
[4]:
fig = df.plot(x="ds", y=["y", "temperature"], figsize=(10, 6))
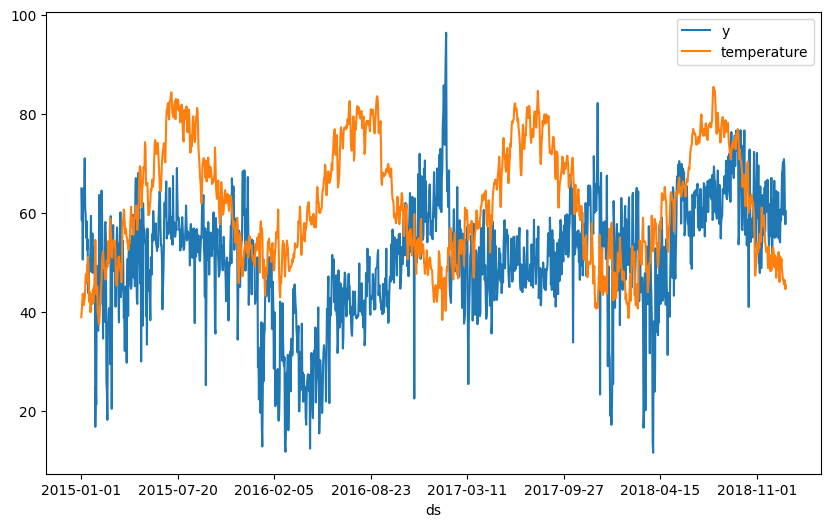
From the data we can see that there is a weak inverse relationship of electricity price to temperature. We start with our model from the previous tutorial and then add temperature as a lagged regressor to our model.
[ ]:
from neuralprophet import NeuralProphet, set_log_level
# Disable logging messages unless there is an error
set_log_level("ERROR")
# Model and prediction
m = NeuralProphet(
n_changepoints=10,
yearly_seasonality=True,
weekly_seasonality=True,
daily_seasonality=False,
n_lags=10, # Autogression
)
m.set_plotting_backend("plotly-static")
# Add temperature of last three days as lagged regressor
m.add_lagged_regressor("temperature", n_lags=3)
# Continue training the model and making a prediction
metrics = m.fit(df)
forecast = m.predict(df)
[16]:
# set plotting to focus on forecasting horizon 1 (the only one for us here)
m.highlight_nth_step_ahead_of_each_forecast(1)
m.plot(forecast)
[15]:
# show the component's forecast contribution
m.plot_components(forecast, components=["lagged_regressors"])
We see that the temperatur impact the forecasted price by few units. Compared to the overall price fluctuations, the temperature impact seems minor, but not insignificant.
[17]:
# visualize model parameters of lagged regression
m.plot_parameters(components=["lagged_regressors"])
The model learns a different weights for each of the lags, which may also capture changes in the direction of temperature.
Let us explore how our model improved after adding the lagged regressor.
[20]:
metrics.tail(1)
[20]:
MAE | RMSE | Loss | RegLoss | epoch | |
---|---|---|---|---|---|
172 | 4.936666 | 6.578746 | 0.00533 | 0.0 | 172 |
[7]:
df_residuals = pd.DataFrame({"ds": df["ds"], "residuals": df["y"] - forecast["yhat1"]})
fig = df_residuals.plot(x="ds", y="residuals", figsize=(10, 6))
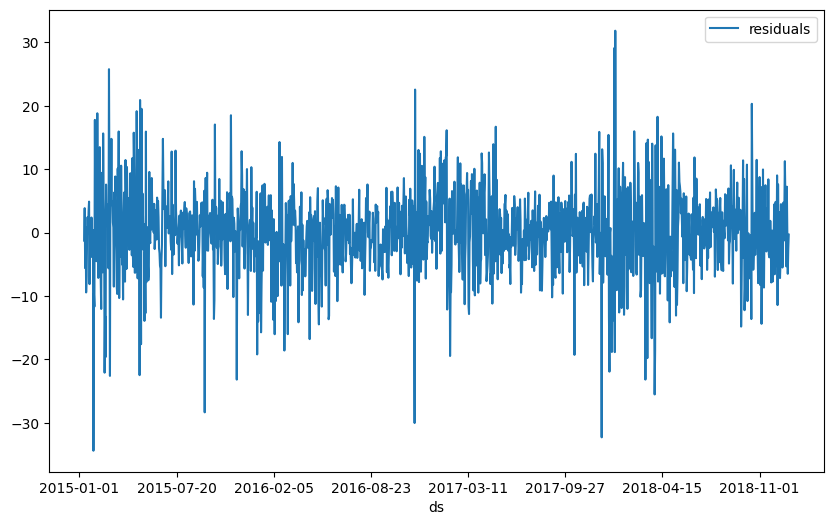